David Kreda, SMART Translation Advisor
Joshua Mandel, SMART Lead Architect
Some readers of our JAMIA paper “Are Meaningful Use Stage 2 certified EHRs ready for Interoperability?” have wondered if we were insinuating that C-CDAs are all but useless because of their heterogeneity and other defects.
We did not say that.
We were definitive only about the express role C-CDAs were intended to serve in Meaningful Use Stage 2. We explained that, from any randomly selected C-CDA document produced by a MU2 certified EHR system, a completely correct and unambiguous reading of all of a single patient’s structured data payload could not happen by software automatically. In truth, even humans would be unable in many cases to disambiguate the statements about the patient for some or all of the data in any produced C-CDA document. The certified system vendors participating in the effort would not have found this at all surprising. Indeed, the vendor feedback at an August session of an ONC Interoperability workgroup affirmed the findings of our paper.
And, thus, we stand by our conclusion: “without timely policy … semantically robust document exchange will not happen anytime soon.”
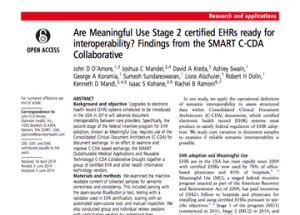
Are Meaningful Use Stage 2 certified EHRs ready for interoperability? Findings from the SMART C-CDA Collaborative
John D D’Amore, Joshua C Mandel, David A Kreda, et al.
J Am Med Inform Assoc published online June 26, 2014
doi: 10.1136/amiajnl-2014-002883
Our recommendations to provide more robust examples, validate codes, and reduce data optionality will reduce variability in the export of C-CDA documents…
Our recommendation to monitor actual C-CDA document exchange would serve vendors well as they write normalizing algorithms. However, even the best engineering cannot reliably populate missing data or resolve conflicting statements, so there will be an upper bound as to what can be normalized. [A] combination of policy changes with community support of our recommendations would move real-world C-CDA exchange closer…
How did we get here? It was nothing sinister, of course, but the combination of small mismatches between the C-CDA Rev 1.1 Standard and the uses planned for it by MU2, incomplete and less than definitive implementation guides, and non-spanning set of certification tests allowed the variability and uncertainties we found. If there is a lesson here, it would be that the process of selecting and applying the use of a standard to get to a highly-specific, quite fault intolerant outcome – semantically interoperable documents – needs precision and coordination in specification, implementation, and testing so that nothing gets lost in translation.
Are we entirely stuck? No. We did not give up on discussing ways to improve C-CDAs. We proposed things – honestly, quite pedestrian interventions – that could be used to improve if not entirely remediate the situation. Crucially, we think many of these fixes can be introduced long before the next round of Meaningful Use certification requirements are realized in production systems.
What, then, are the uses for C-CDA documents? Within provider organizations, where security and privacy are comparatively well constrained, C-CDA documents are likely to be useful for data mining of all types. The ambiguity we have described in the MU2 use case will matter far less for “secondary uses” of the data. For example: automatically analyzing C-CDAs for epidemiological trends, service trends, quality trends, etc. are well within reach. Or, as we recommended, evaluating the quality of medical record by checking the C-CDA would itself be a good idea. It should be possible to look for stale data, mismatches between structured and unstructured data, etc., with one worthwhile goal being to flag records which need to be cleaned up! Another especially promising use case for C-CDA would be to “fuel” advances in predictive analytics. Though C-CDA documents are not explicitly discussed, a recent paper by Bates and colleagues in Health Affairs identified six areas (high-cost patients, readmissions, triage, decompensation, adverse events, and diseases affecting multiple organ systems) where developing effective predictive analytics could deliver great value for healthcare.
You must be logged in to post a comment.